Application of genetic algorithms in iGaming development
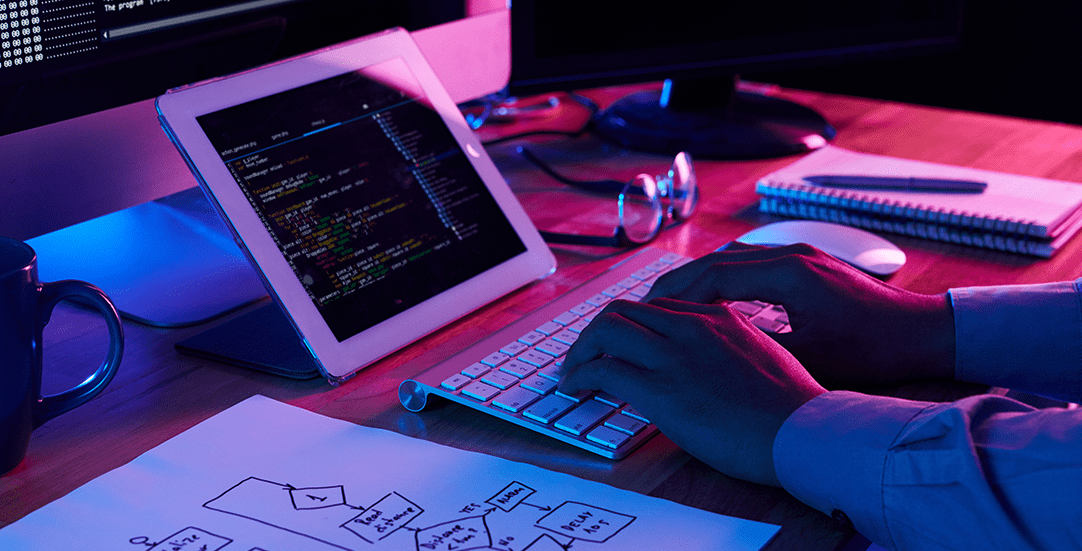
Understanding the crux of genetic algorithms opens a plethora of possibilities for pushing the envelope in iGaming development. Bridging the gap between complex computations and gameplay, genetic algorithms hold the key to a more immersive and dynamic iGaming experience.
Demystifying genetic algorithms in iGaming
Genetic algorithms trade on the principles of natural selection, which, in the realm of iGaming, translates into creating more engaging games that can adapt and evolve. They work by simulating a population that evolves, with evolution steered by factors such as mutation, crossover, and selection.
They can be applied to optimize game parameters and mechanics, creating an environment that continually adjusts and enhances itself based on player behavior and preferences. This results in games that are highly personalized and immersive, offering unique experiences for each player.
Below we will present an example of a scenario that can be used in slots.
Unveiling the core concepts of genetic algorithms
In the specific context of a slot machine game, every one of the genetic algorithms represents a possible configuration of symbols on the reels. Each symbol positioned on a reel is encoded as an integer, signifying that both the symbol type and its specific position are significant parameters in the configuration. The initialization of the population is done by introducing random variations (noise) to an initial reel’s configuration.
The ‘fitness’ of an individual is determined using a fitness function unique to the problem at hand. In the slot machine’s case, the difference between the desired Return to Player (RTP) and the actual RTP obtained from the configuration is used as the fitness function. Monte Carlo simulations are employed to estimate the RTP over many separate runs. The aim of this solution in this context is to evolve reel configurations such that this difference is minimized.
Refining slot machine reels configuration with genetic algorithms
This branch of machine learning operates through iterative generations. Each generation involves the selection of parent individuals based on their fitness scores, the crossover (or recombination) of these parents to create offspring, and the mutation of offspring to introduce variability.
In this selection process, balanced consideration is given to the fittest solutions and a random subset of the population, ensuring a measure of diversity. This method discourages premature convergence to a sub-optimal solution.
The process of crossover involves recombining attributes of two-parent individuals to create an offspring configuration. Here, a single-point cut is used, meaning that elements from each parent are combined at a particular cut point to generate new solutions. The concept of using more than two parents for recombination can be explored in further research to determine if it could obtain better results.
Mutation is introduced after crossover to maintain diversity within the population and avoid premature convergence to local optima. It involves a random alteration of selected symbols on the slot reels, substituting one symbol for another.
The algorithm continues iterating through these genetic operations across multiple generations. The inclusion of an elitist rule ensures the survival of the most optimal solutions from one generation to the next, preserving valuable characteristics.
Determining the termination of the algorithm
The termination criteria for this kind of computational technique revolves primarily around reaching a stipulated maximum number of generations. Additionally, human involvement is permitted in guiding the process – either through observation or by directly intervening to halt the algorithm.
Upon termination, the outcome is an integer vector that mirrors a slot machine’s symbolic layout, optimized to achieve an RTP as close as possible to the specified objective. For instance, if a slot game layout consists of five reels (displayed in five columns and three rows), with each reel containing 63 symbols, the computational solution would depict a configuration framework of shape 5×63.
This sophisticated application of these algorithms provides an insightful look into their capabilities and distinct elegance in refining complex iGaming scenarios, like aiming for an ideal RTP in slot machines.
Understanding the role of the configuration matrix
In the intricately digital landscape of online gaming, slot machines carry the charm of being seemingly simple yet profoundly enigmatic. They lure in patrons with their colorful reels rich in a variety of symbols. However, beneath this vibrant veneer, lies an intricate configuration, a ‘map’ determining the game outcome. This configuration is diligently optimized to closely align with a desired RTP rate.
The powerhouse driving this optimization? The unassuming matrices in tandem with genetic algorithms. A slot machine configuration can be visualized as a matrix, with each row representing a symbol position and every column embodying a reel on the machine. The intersection of a row and a column determines which symbol resides at a specific position on a given reel.
To illustrate, let’s consider a simplified scenario: a slot machine game with five reels, each bearing just five symbols.
In this sample matrix, the symbol and its position on each reel are clearly denoted. Note that the real-world slot machine configuration matrix would be far larger – typically 5×63, encompassing a wide variety of symbols on each reel.
Thus, matrices offer an elegant and tangible means of encapsulating a slot machine’s complex symbol configuration. From this structure, the algorithm can undertake iterations, selections, cross-over, and mutation operations to incrementally refine the machine’s performance. The outcome is a meticulously calibrated configuration that streamlines the machine’s RTP towards its preferred value. This not only exemplifies the profound synergy between gaming and mathematics, but also illustrates the remarkable effectiveness of these evolutionary algorithms in building a captivating casino experience.
Refining iGaming experiences with genetic algorithms
Chemistry and the power of this kind of machine learning solution can bring groundbreaking advancement in iGaming gamification and player experience. They can fine-tune gameplay tactics and generate engaging content dynamically.
Enhancing iGaming tactics with genetic algorithms
In iGaming, there are often complex scenarios where results hinge on strategic decisions made by players. This is particularly true in games like online poker, blackjack, or other games that mix elements of strategy and luck.
A perfect strategy for these games can be incorporated into an advanced algorithm where each part of the algorithmic structure signifies a specific decision for a particular game situation. Computational operations are performed iteratively on a pool of these strategies. The strategies’ effectiveness is evaluated using a fitness function, typically the accumulated earnings in simulated games.
Over multiple iterations, these advanced algorithms can contribute to the development of strategies that enrich the gameplay experience. This enhancement makes games more strategically diverse, challenging, and captivating.
Procedural content generation
Procedurally generated content is a potent force in driving dynamic and unique gaming experiences. Evolutionary algorithms form the essential support system enabling meticulous augmentation of these procedural generation mechanisms. The fitness functions assess aspects such as the fairness of the content generated, the balance in gameplay dynamics, how effectively it fosters engaging player interactions, and its influence on maintaining player interest.
Drawing parallels to the evolution of strategies, these specialized operations are cyclically exercised on a range of content generation methods. They evolve gradually to finely tune into player preferences, assuring an immersive and unique iGaming experience at every encounter.
Genetic algorithms in player engagement
This type of machine learning algorithms, by tailoring gambling experiences and promoting sustained interest, are a potent tool to boost player engagement in the iGaming industry.
Personalizing player experiences
In the context of iGaming, each player interacts with the game in their own way. Genetic algorithms can capture these nuanced interactions and mold unique experiences tailored for everyone.
The algorithm progressively learns player preferences regarding specific game aspects like interfaces, difficulty levels, or game flow mechanics and optimizes these elements uniquely for each player. The progressive iterations and adaptability of the algorithm yield a customized gaming environment, enhancing immersion and satisfaction for players.
Retaining user interest
In the long run, retaining player interest is vital for the success of an iGaming platform.
For example, in designing online casino games that engage multiple variables, genetic algorithms can facilitate the efficient creation of newer games with more engaging rules, payouts, and challenges. This process can be guided by using insights gleaned from individual player behavior and feedback.
Leveraging genetic algorithms for robust iGaming platform
The vast expansion of internet and communication technologies has revolutionized the way we engage with digital platforms, including iGaming. However, this rapid transformation has brought along novel cyber threats, making the security of these platforms more critical than ever. Genetic algorithms, in tandem with other cybersecurity tools, offer a promising approach to effectively safeguard our digital arenas.
Bolstering security measures with genetic algorithm tool
Evolutionary computation can hold a crucial role in bolstering cybersecurity mechanisms of iGaming platforms – a space where data safety, along with user confidence, is of utmost importance. Traditional defenses like firewalls and antivirus software offer foundational security. However, Intrusion Detection Systems (IDS), enhanced with computational techniques, provide a more advanced protective layer.
These detection systems are tasked with identifying unusual behavior, unauthorized network access, and potential threats. Despite the critical role IDS plays in ensuring network security, they encounter multiple challenges, such as a lack of autonomy in neutralizing threats, triggering false alarms, plus the necessity for dedicated professionals for their operational management. Evolutionary computational techniques can address these issues, enhancing their efficiency through machine-learning-based decision-making, thereby reducing false alarms and administrative workload.
In the iGaming sector, these advanced detection systems can identify deviations from expected player behavior, flag suspicious activities, and alert security professionals in real-time. By optimizing these mechanisms, these algorithms can aid IDS in recognizing and countering a wide range of threats and attempted breaches.
Refined risk management in casino systems
These computation techniques have a remarkable aptitude for being applied to analyze player behavior, pinpoint patterns that deviate from the norm, and swiftly isolate anomalies. By continually learning and evolving, the technique can adapt to emerging illicit tactics and persistently sharpen its intrusion detection capability.
These models excel in identifying zero-day attacks, where unknown system vulnerabilities are exploited by hackers – a growing concern in today’s cyber landscape. Through rapid detection of these breaches, evolutionary computation can help reduce potential damage and ensure players’ trust in the online casino platforms remains unscathed.
Risk management in casino platforms can extend not only to securing the system but also to modeling financial risk, detecting potential fraud, and optimizing payout structures to sustain profitability while ensuring player engagement. In these sophisticated settings, the algorithms, with their inherent ability to optimize multifaceted systems, can prove invaluable.
For those seeking a more in-depth exploration of using genetic algorithms for network security, the research paper titled Robust Genetic Machine Learning Ensemble Model for Intrusion Detection in Network Traffic is the right answer.
Driving future trends in iGaming with genetic algorithms
Computational techniques, such as evolutionary algorithms, serve as guiding lights for the progress within the iGaming industry. Using the power of these optimization mechanisms can result in notable improvements across various facets of iGaming. They can be utilized to craft deeply personalized gaming experiences, fine-tune gameplay mechanics, and generate procedural content that maintains the freshness of every gaming session.
In addition, these models contribute to the effective management of risks in casino systems. However, it’s essential to note that while they offer immense potential, evolutionary algorithms are not a blanket solution. Different scenarios may warrant other specific strategies or techniques for optimal outcomes.
However, they show promise in adapting to future trends, shaping, and redefining the future of the iGaming industry. While they are a substantial force helping to navigate the seas of progress, it’s crucial to explore and employ a multitude of methods in this dynamic field. As the saying goes, there is more than one way to roll the dice.
Feel free to reach out to us with any queries or for personalized advice. Our team of experts is always ready and eager to assist. Visit our contact page and let’s roll the dice on the future of iGaming together.